- | Technology and Innovation Technology and Innovation
- | Policy Briefs Policy Briefs
- |
Aggregated Smartphone Location Data to Assist in Response to Pandemic
The sudden, mandated closures of businesses, restaurants, and schools in response to the COVID-19 pandemic impose severe economic and social costs. People want to know when they can reopen their shops, rehire furloughed employees, and return to a life with some semblance of normalcy. Medical researchers currently estimate that the use of hospital resources will peak and ebb in most of the country in two or three weeks (mid-April to late April). Once there is slack in hospital resources, many people will be eager to return to work, worship, travel, and recreation.
Given the rapid transmission of COVID-19 and its severe health effects, stay-at-home rules and norms should be relaxed only in locations where the risk of infection is low. Data on where stay-at-home norms are practiced can help public officials identify the counties and regions where residents can go back to work and where resources (such as testing kits or public service announcements) should be allocated in order to minimize the chance of another surge of infection.
On March 26, the president announced pending guidelines in a letter to governors, including a nationwide effort to “[categorize] counties as high-risk, medium-risk, or low-risk” for COVID-19 spread. We explain below how aggregated, anonymized smartphone data can serve as a powerful analytical tool for policymakers in their attempts to control COVID-19 infection while freeing households in low-risk regions and neighborhoods from stay-at-home mandates and business restrictions.
Using Anonymized Location Data to Help Categorize High- and Low-Risk Areas
For the first time in history, most American adults carry an always-on geolocation device—their smartphone—on their person most of the time. Many popular smartphone apps passively transmit user location—via cellular, GPS, WiFi, or Bluetooth technology—to app companies at all hours of the day. These millions of data points are used by app companies to target users with local ads or to improve proximity-based services such as dating apps, mapping apps, or recommended restaurants.
These are rich datasets that public health officials and researchers are using to evaluate shelter-at-home compliance and model COVID-19 transmission speeds. For example, officials in Austria and Italy are already using anonymized location information from mobile phone company records to examine the efficacy of their COVID-19 lockdowns.
Similar analysis is underway in the United States. According to the Washington Post and Wall Street Journal, large and small smartphone app companies are sharing aggregated, anonymized location information with the Centers for Disease Control and Prevention (CDC) to help combat the spread of COVID-19. Some analysis is shared publicly. On March 24, Unacast, a company that aggregates and analyzes user location data, launched a “Social Distancing Scoreboard” that examines how residents in each US county are reducing their travel in response to the pandemic. The Scoreboard ranks both counties and states on a scale from A through F.
Privacy Issues
Health officials should avoid examining specific individuals, but rather analyze aggregated data to make accurate generalizations about whether a neighborhood, town, county, or state is engaging in contagion-limiting practices. This analysis will allow authorities to prioritize the disbursement of scarce medical resources and assess the efficacy of different policies. By all indications, tech companies are sharing geolocation with public officials voluntarily, which means there’s unlikely to be a Fourth Amendment problem—information that is voluntarily shared does not constitute a government search or seizure.
That said, in addition to making location data anonymized and aggregated, any data-sharing agreement between data providers and government officials should have a firm end date (with extensions as needed) so that once the pandemic crisis passes, public officials are not accessing sensitive location data in nonemergency circumstances. In addition, data-sharing agreements should be solely with agencies focused on public health, such as the CDC and state health offices, and should be restricted from use by law enforcement. Though it is more protective of user privacy preferences, an “opt-in system”—whereby public health researchers examine only the movements of users who affirmatively download an app designed by public health researchers—will not, we think, be as effective as crowdsourcing data collection from various commercial apps.
Step 1: Categorize Different Areas Based on Their Infection or Hospitalization Rates and “Social Distancing” Practices
Public health officials should evaluate location trends based on the location of medical facilities that report COVID-19 infections—perhaps at the census-block or ZIP-code level. Reporting based on, say, the county level might provide an inaccurate picture because many counties lack medical facilities that report infection and hospitalization data.
If most residents are spending 22 hours in one location except for brief visits to the grocery store and parks, that is a reliable sign that people are engaging in fairly strict social distancing behaviors. If, on the other hand, resident commuting and travel is common, then that area is likely engaging in weaker social distancing behaviors.
Combining these data with reported infection and hospitalization rates will allow policymakers to analyze various jurisdictions and geographic areas based on a matrix of risk levels (see table 1).
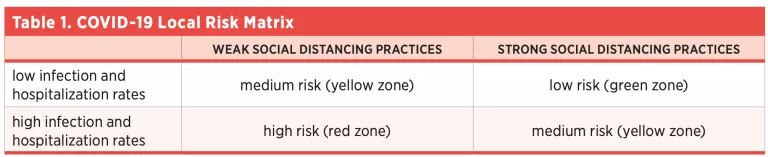
This matrix should enable policymakers to prioritize resources for strict social distancing enforcement, testing, and loosening of stay-at-home orders. Promulgating ascertainable criteria—say, no new reported cases in the past Nweeks—and pairing those criteria with social distancing measurements will allow residents and authorities to anticipate and declare an easing of local stay-at-home orders.
Step 2: Combine Risk-Matrix Data with Testing Data to Support Crisis Management
The next step in the process is for policymakers and health officials to tailor their responses to the needs of the specific area they are governing based on which category that area falls into.
Where infection and hospitalization rates are low and location data indicate that people are practicing strict distancing, COVID-19 testing is the area of emphasis. As soon as their production ramps up, tests should be distributed widely in areas that are low risk: that is, they have low infection rates and a good social distancing score. This approach will achieve two things: (1) infected, asymptomatic people who might unintentionally spread the disease are informed about the need to stay home, and (2) uninfected people can start returning to work and a somewhat normal life with some level of confidence that the other individuals they meet will not be contagious.
The identification of green zones could be an important data point for epidemiologists and other public health authorities managing the crisis to consider relaxing harsher social distancing enforcement policies and let people gradually return to a broader array of activities. This approach can help alleviate some of the costs that come with intense social isolation. That said, relaxing social distancing enforcement policies should be done with local circumstances in mind. For instance, loosening stay-at-home orders should be slower in areas that are transportation hubs, that are professional conference locations, or that otherwise raise the risk of large numbers of nonlocals meeting in close proximity.
On the other hand, in red zones, where infection and hospitalization rates are high and people are not strictly distancing, there is a stronger need for increased public education and, potentially, stricter social distancing enforcement. These are areas where minimizing human contact is of the utmost importance, and they will likely require more aggressive public-sector intervention.
In the foreseeable future, the vast majority of the country will remain in yellow zones. In those localities, many more factors will need to be considered for a change of course in the way the crisis is being managed, with special attention to the conditions on the ground and hospital capacity. However, this framework should at least allow for a refocusing in green and red zones, respectively.
Conclusion
The COVID-19 epidemic has disrupted American business, education, and home life. Once peak load on hospitals diminishes, public officials and residents will want to return to their normal lives. Public health officials and policymakers across the nation will likely begin categorizing counties as having a low, medium, or high risk of infection when they consider the end of stay-at-home orders and norms. Smartphone geolocation data can provide public health officials useful information for determining county and regional risk criteria. App companies that volunteer these data should scrupulously use anonymization and aggregation, as well as other controls in place. Public health surveillance can be a useful tool for restoring commercial and social life in many American cities and towns, but app companies should assist the public effort with user privacy in mind.
About the Authors
Brent Skorup is a senior research fellow at the Mercatus Center at George Mason University. His research areas include transportation technology, telecommunications, aviation, and wireless policy. He serves on the Federal Communications Commission’s Broadband Deployment Advisory Committee and on the Texas Department of Transportation’s Connected and Autonomous Vehicle Task Force. He is also a member of the Federalist Society’s Regulatory Transparency Project.
Trace Mitchell is a research associate at the Mercatus Center at George Mason University. Previously, he has interned with the Mercatus Center’s Academic and Student Programs team. Mitchell is a graduate of Florida Gulf Coast University, where he received a BA in political science. He is currently pursuing a JD at George Mason University’s Antonin Scalia Law School. He is also an alumnus of the Mercatus Center’s Frédéric Bastiat Fellowship.