- | Healthcare Healthcare
- | Policy Briefs Policy Briefs
- |
Healthcare RegData: Quantifying the Volume of Healthcare Regulations
Regulations have played a significant role in shaping the healthcare industry in the United States. Through financing healthcare, licensing healthcare practitioners, educating healthcare professionals, establishing and operating healthcare facilities, and controlling the nature of the care to be provided, among other functions, federal and state healthcare regulations have over time created the complex US healthcare system of today.
While researchers over the years have examined the impact of specific regulations on various outcomes, they have paid very little attention to the volume or other quantifiable characteristics of healthcare regulations. This is because the sheer volume of regulations makes them difficult to analyze, and their format—text—calls for special tools to examine them. We aim to address this deficiency in data availability by employing the QuantGov and RegData platforms, developed by the Mercatus Center at George Mason University, to quantify the volume of healthcare regulations in the United States, including both federal and state regulations. In addition, we examine the industries that are most affected by healthcare regulations, and we measure the complexity of the regulations.
Since 2012, the Mercatus Center’s RegData project has made it possible for policymakers and stakeholders to discuss the effects of regulations by providing a quantifiable and replicable way of measuring regulations in a growing number of national and subnational jurisdictions, including the United States, Canada, and Australia. RegData measures the volume of regulations by counting the number of restrictions found in a unit of regulation.
By design, regulations impose restrictions on an agent of society by either preventing or mandating specific activities. Therefore, RegData quantifies the number of regulatory restrictions by counting the number of restrictive terms in a unit of regulatory text. In RegData, a regulatory restriction is defined as the occurrence any of the following terms, which are commonly associated with regulatory restrictions, in a unit of regulation: “shall,” “must,” “may not,” “required,” and “prohibited.” In addition to counting the number of restrictions, RegData also uses QuantGov, a machine learning platform created by the Mercatus Center, to estimate the probability that the text of a regulation applies to a specific industry. This unique feature of RegData allows for the examination of the impact of regulations on specific industries.
Healthcare RegData extends the RegData approach to analyze healthcare regulations in the United States. The publication of this brief and the accompanying data marks the beginning of a long-term project to quantify the volume of healthcare regulations and the evolution of healthcare regulation since 1996. This first iteration of Healthcare RegData examines the stock of healthcare restrictions as of December 2018. The main source of data is the US Code of Federal Regulations(CFR), published by the Government Publishing Office.
Methodology and Data
The CFR, which contains all US federal regulations, is organized into 50 titles, each of which covers a broad subject area. Each title is subdivided into parts, and parts are divided into sections. (Some large parts have subparts before sections.) In Healthcare RegData, we evaluate regulations at the section level. In order to accurately identify regulatory restrictions, it is critical to determine the unit of analysis and the definition of a healthcare regulation. A unit of regulation is determined to pertain to healthcare if it concerns the provision of healthcare goods and services. Using this narrow definition, we exclude regulations that concern food, alcohol, air quality, labor conditions, and so forth, which may have the goal of improving the health status of Americans but do not directly relate to the provision of healthcare goods and services. Under this narrow definition, regulations related to pharmaceutical drugs are considered to be healthcare regulations. For Healthcare RegData, we chose CFR sections to be the unit of analysis. This means a section is considered a unit of regulation.
A team of researchers reviewed all the CFR titles to identify parts and sections that regulate healthcare. They then extracted these regulations from the CFR and analyzed them using the QuantGov platform. This approach of manually identifying regulations is naturally prone to human error. However, it provides a reasonable approximation of the total number of federal healthcare regulations. In subsequent versions of Healthcare RegData, we will use the QuantGov platform to train an algorithm to identify healthcare regulations at both the federal and state levels.
In addition to counting regulatory restrictions, RegData also determines the industries that are likely to be affected by a unit of regulation. We use the North American Industry Classification System (NAICS) codes to define the industries. We then use RegData’s machine learning algorithms to classify regulations into industries by assigning a probability that a unit of regulation applies to an industry identified by a NAICS code. Using the probabilities and the total number of restrictions identified in the unit of regulation, we derive the industry-relevant restrictions, which is the total number of restrictions multiplied by the probability that the unit of regulation applies to an industry.
Finally, RegData allows us to examine the complexity of regulations. We use the term complexity to capture a few concepts related to compliance with regulations. These include the readability of the regulatory text and the ease of comprehension. The ease of reading and comprehending the regulatory text is important because, all else being equal, text that is easy to read and comprehend will have lower compliance costs than text that is more difficult to read and understand. As stated in the Federal Plain Language Guidelines of the US government, complex ideas are easier to grasp when they are presented in a manner that adheres to plain-language principles such as using short sentences and few conditional clauses.
Using the CFR data, RegData provides a number of metrics to quantify the complexity of healthcare regulations. These are the unit of regulation’s readability (using the Flesch Reading Ease Score), the average sentence length, and the number of conditional statements in the unit of regulation. In addition, we borrow a concept from information theory known as Shannon entropy. Shannon entropy simply measures the rate at which new information is introduced in a unit of text. If a lot of new information is introduced, the text is more difficult to read and understand.
The Volume of Federal Healthcare Regulations in the United States
From the 2018 CFR, we identified 49,312 healthcare regulatory restrictions as of December 2018, comprising 4.5 percent of all federal regulatory restrictions (1,085,063). As one would expect, the Department of Health and Human Services (HHS) has issued 85.4 percent of all healthcare regulatory restrictions (42,088). Table 1, ordered from the agency that has issued the most healthcare restrictions to the one that has issued the least, shows that five agencies—mostly executive departments—have issued 98.5 percent of all federal healthcare regulatory restrictions. For comparison, table 1 also shows how many restrictions of any kind (including healthcare restrictions) these same agencies have issued.
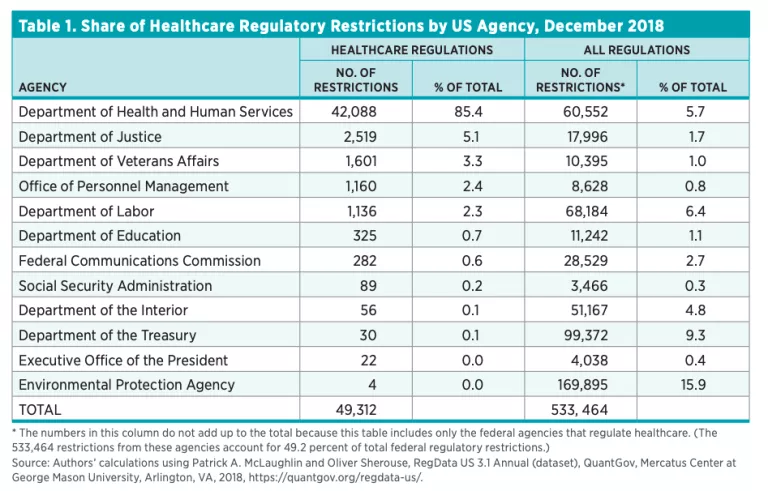
Table 2 shows the total restrictions issued by offices within HHS. As one would expect, the Centers for Medicare and Medicaid Services and the Food and Drug Administration have issued most of the healthcare restrictions.
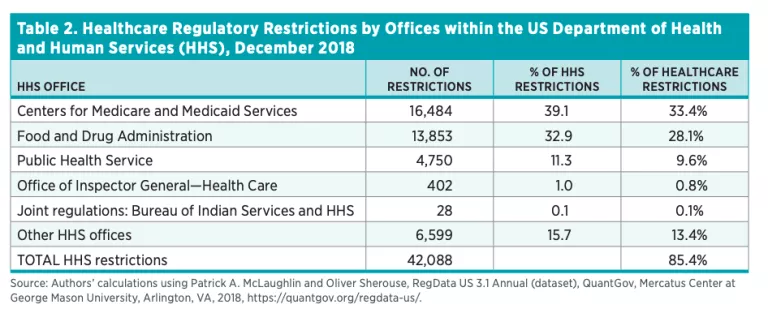
Table 3 shows the industries affected by the healthcare regulations identified, ordered by the number of industry-relevant restrictions. Industry-relevant restrictions, as mentioned previously, are the predicted probability that a unit of regulation affects an industry multiplied by the total number of regulatory restrictions in that unit. As one would expect, the industry most affected by healthcare regulations is ambulatory healthcare services. Industries that are directly associated with healthcare, such as hospitals and insurance providers, are in the top 10.
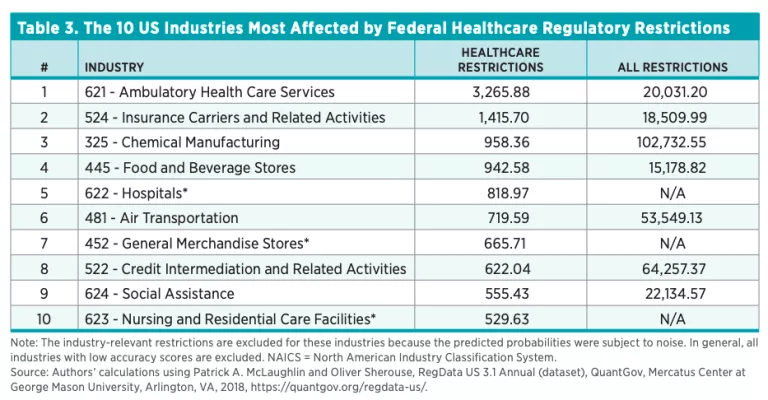
Complexity of Regulations
RegData also provides tools to analyze the language used in writing regulations. As explained in the methodology and data section, we examine the complexity of healthcare regulations using three measures of complexity from the QuantGov platform. These are the average reading ease, the average sentence length, and the Shannon entropy score. In table 4, we show the averages of reading ease, sentence length (number of words), and Shannon entropy for US federal healthcare regulations. The reading ease measure uses the Flesch Reading Ease formula, which scores a unit of text on a scale up to 100. Generally, the higher the Flesch score, the more readable the text. There is no lower limit for the score, so it is possible for a unit of text to receive a negative score, and indeed many healthcare regulations issued by HHS do receive negative scores (see table 4). As a rule of thumb, documents with scores between 80 and 100 are considered easy to read. Documents with scores under 60 are considered difficult to read.
Table 4 shows that the average federal regulation is quite difficult to read and readers require high levels of education and subject-matter expertise to understand it. The average, however, masks significant differences across agencies. For example, HHS, which issues more than 85 percent of all healthcare regulations, has a Flesch score of −2.4, compared to scores of 35.5 and 25.1 for the Department of the Treasury and the Executive Office of the President, respectively. In general, the average sentence length correlates with the Flesch score, because the formula for the Flesch score considers average sentence length.
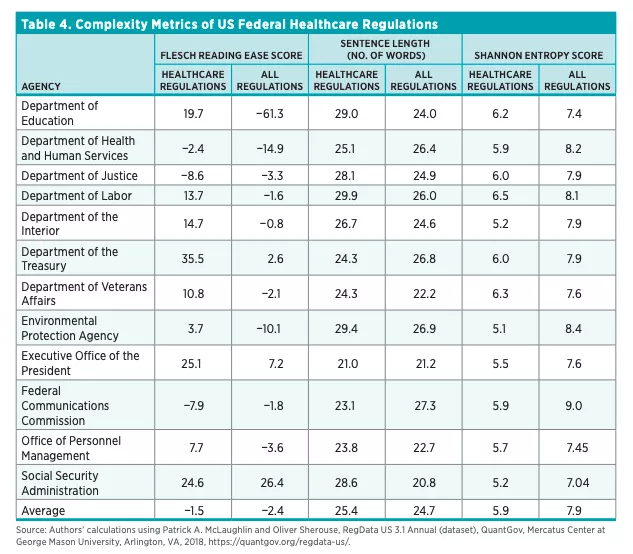
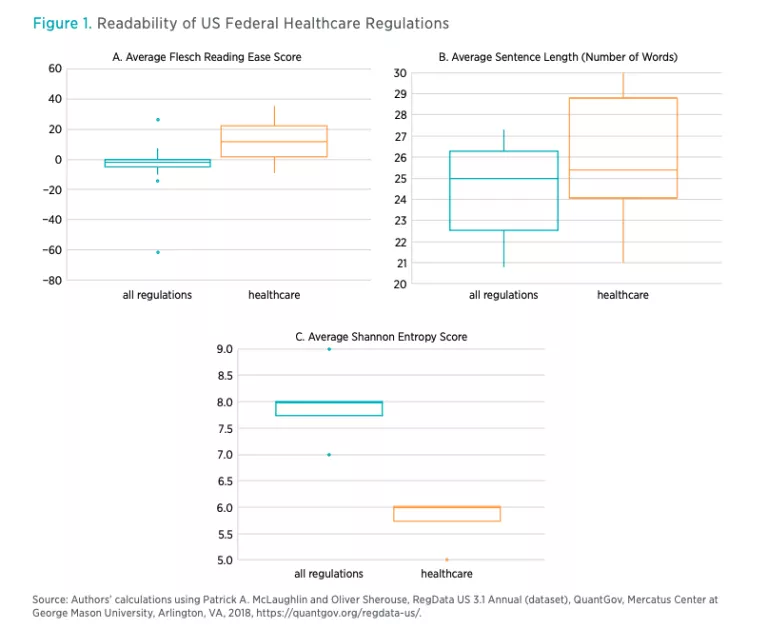
Conclusion and Next Steps
Healthcare regulations have played an important role in shaping the healthcare sector over the years. Healthcare RegData is an attempt to quantify the volume of healthcare regulations and their impact on the healthcare industry and health outcomes. Unsurprisingly, the Centers for Medicare and Medicaid Services within HHS has issued the most healthcare regulations—33 percent, followed by the FDA and the Public Health Service with 28 percent and 10 percent respectively. The industries most impacted by these regulations are ambulatory healthcare services, insurance carriers, and chemical manufacturing.
In this first edition of Healthcare RegData, we have provided a snapshot of the volume of healthcare regulatory restrictions. This is merely the first step and the baseline for subsequent analyses. Over the next few months, we will examine the growth of healthcare regulations from 1996 to the present. In addition, we will examine the volume of healthcare regulations in the states and classify these regulations into various topics of interest. Some of these topics include occupational licensing, certificate-of-need laws, and public health. These analyses will allow researchers to examine the role of healthcare regulations in shaping the evolution of healthcare in the United States. In addition, the data on the complexity of regulations should be useful to legislators and regulators as they create or manage regulations.