- | International Freedom and Trade International Freedom and Trade
- | Policy Briefs Policy Briefs
- |
Predicting Taiwan Strait Crises Using Propaganda: A New Open-Source Method
LLMs help read between the lines of China's daily newspaper
In the Chinese Communist Party (CCP)’s eyes, the question of what to do about Taiwan—officially the Republic of China, which is separate from the CCP-ruled People's Republic of China (PRC)—is arguably one of the most existential policy issues for Beijing. The CCP has long viewed Taiwan as a renegade province that should be returned to the PRC—by force if necessary. For the CCP, “reunification” with the island is not a matter of if, but when.
But when? That’s anyone’s guess. US military leaders, for example, have differing estimates of when a Chinese invasion of Taiwan might occur: A former head of the Indo-Pacific Command predicted it would happen by 2027, while a former commander of the Air Mobility Command only gave it only until 2025—or what remains of it. A former Chief of Naval Operations even thought it would happen in 2022 or 2023.[1] Other aspects of a potential conflict are just as uncertain: whether it will be a full-scale invasion, whether the PRC can win, and how the US will be involved.[2] After all, a Chinese invasion of Taiwan would be an unprecedented event—it has never happened before—even though multiple Taiwan Strait Crises in the past have brought the two sides close to it.
In this policy brief, we introduce a new open-source method that uses large language models (LLMs) to analyze CCP propaganda and develop early warning signals for a Taiwan Strait crisis—potentially a prelude to an invasion. In 2018, Weifeng Zhong co-created the Policy Change Index (PCI) project for China, which predicts Beijing’s policy moves by analyzing People’s Daily, the CCP’s most prominent official newspaper.[3] This study builds on that approach but focuses on a more specific issue.
The Value of Propaganda
Government propaganda in authoritarian regimes is often, and rightfully, criticized for containing biases and disinformation and for failing to meet journalistic standards. For this reason, researchers often avoid using propaganda as a source of information.[4] However, dismissing its value altogether would be misguided. In fact, propaganda content is exceptionally useful—not as a reflection of truth, but as a tool for making inferences about a regime’s views and intentions.
The inferential value of propaganda can be traced back to World War II. In the fall of 1943, Allied reconnaissance discovered a series of discreet German installations along the Nazi-occupied French coast. The Allies watched the construction with great anxiety, as it appeared to be a network of secret weapon sites intended for attacking London—the world’s most populous city at the time. An opposing view within the Allied forces, however, held that the installations were a Nazi hoax designed to divert Allied attention and resources.
Fortunately, the first view prevailed, and the Allies carried out numerous bombardments to destroy what would have otherwise become firing sites for the V-1 flying bomb. According to Dwight D. Eisenhower, the Allies’ supreme commander in Europe at the time, had they made the wrong call on these weapon sites, Operation Overlord might have been written off.[5]
Among those within the Allied forces who correctly assessed the situation were propaganda analysts whose job was to make inferences about the Nazis by reading between the lines of German propaganda. As documented in the book Propaganda Analysis by political scientist Alexander George, 81 percent of the inferences made by American propaganda analysts about the Nazis during the war proved to be correct.[6]
Nazi propagandists didn’t openly share their secrets on the radio, of course. Yet their secrets were revealed because their propaganda was a product of multiple factors of great interest to the Allies: the Nazi regime’s situation, its intended actions, and the goals its propaganda aimed to achieve, among others. Since these factors influenced propaganda content, “reverse-engineering” it allowed Allied analysts to better understand them.
The same logic applies to CCP propaganda, which has remained consistently disciplined throughout the history of the PRC. People’s Daily, the most prominent party mouthpiece, has been the Chinese government’s primary platform for promoting its viewpoints and communicating its directives to the rest of the country—including whether and when it intends to escalate toward Taiwan. Leveraging this disciplined publication, the PCI project has demonstrated that when the newspaper shifts its focus, it often signals the party’s subsequent changes in policy priorities.[7]
The Policy Change Index for Tensions (PCI-Tensions)
Capitalizing on the inferential value of CCP propaganda, we have developed the Policy Change Index for Tensions (PCI-Tensions), which uses an LLM to track the ebb and flow of tensions toward Taiwan in People’s Daily. We hypothesize that when tensions are elevated in the newspaper, a potential crisis—or even a kinetic conflict—between China and Taiwan becomes more likely.
PCI-Tensions Framework
Our approach involves two phases. In the first, training phase, we develop an artificial intelligence model using People’s Daily articles published before and during the 1995 Taiwan Strait Crisis, which led to military escalations by China, Taiwan, and the US before subsiding. In the second, test phase, we assess the model’s performance on the recent cross-Strait political events from 2022 to 2024, including major visits and meetings between US and Taiwanese leaders, some of which were associated with higher cross-Strait tensions than others.
We took the following steps to develop the PCI-Tensions algorithm.[8]
- Collect the full text of the People’s Daily from the 1994–1996 training period and 2022–2024 test period and label a set of essential metadata for each article, such as publication date, title, content, and page number.
- Identify major events that occurred leading up to and during the 1995 Taiwan Strait Crisis (covered by the training period) and recent US-Taiwan diplomatic events in the test period.[9]
- For each newspaper article in the training period, build a set of quantitative indices to measure China-Taiwan relations by prompting an LLM with questions such as how the relationship between China and Taiwan is perceived and how the Chinese government views the Taiwanese government.[10] Then, aggregate each article-level index to a weekly sum and calculate the four-week moving average of that sum.[11]
- Assess how well the time series of the indices match or even predict the timing of the major events during the 1994–1996 period.
- Fine-tune the algorithm by repeating steps 3 and 4. Specifically, improve the indices by revising the LLM prompts to better capture a variety of topics most relevant to China-Taiwan tensions, such as military activities, US engagement, economic relations, culture exchange, and China’s emphasis on reunification and the One China principle. The goal of this step is to optimize the fit of the indices to the timing of the major events.
- Assess the model’s performance by deploying the model to People’s Daily articles in the 2022–2024 period, which covers major political events, including Taiwan’s diplomatic activities and China’s military escalations, across the Taiwan Strait.[12]
Figure 1 plots the weekly PCI-Tensions between China and Taiwan for the 1994–1996 training period (the left column) and the 2022–2024 test period (the right column) across a set of four key LLM prompts. In the figure, we also overlay the timing of major events on the indices for comparison. Each of the four indices represents a rating from People’s Daily’s perspective on a key topic:[13]
- China’s attitude toward Taiwan (on a scale from –1 to 1)
- China’s attitude toward the US (on a scale from –1 to 1)
- Prospect of military activities between China and Taiwan (on a scale from 0 to 1)
- Prospect of China’s reunification with Taiwan (on a scale from 0 to 1)
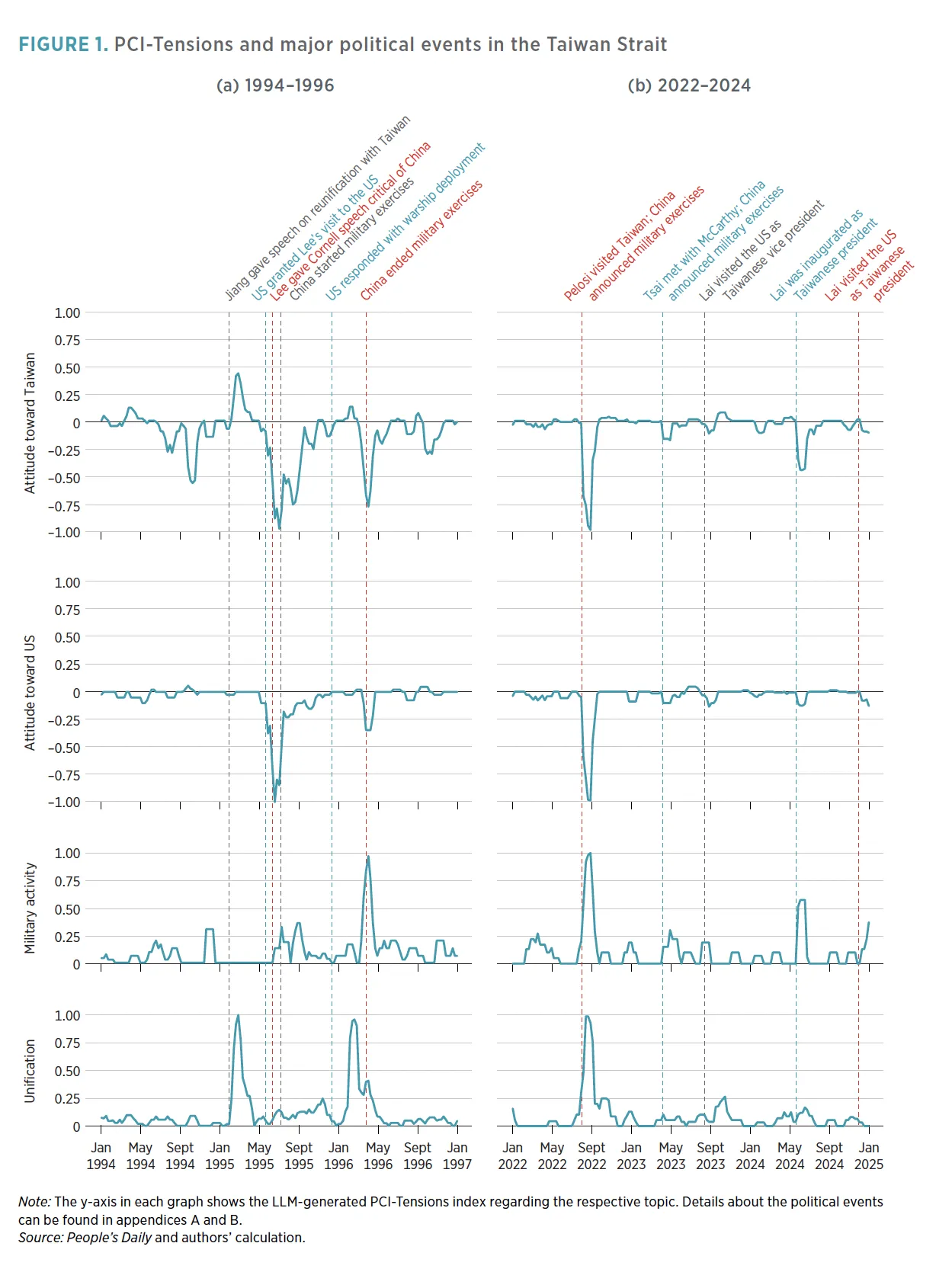
As the left column of plots shows, the 1995 Taiwan Strait Crisis, which started on May 22, 1995, and ended on March 25, 1996, was associated with a declining Chinese attitude toward Taiwan and the US (the first two indices), as well as heightened prospects of cross-Strait military activities and China’s reunification with Taiwan (the last two indices). Moreover, such movements in the indices tend to precede the onset of the crisis, suggesting that the former could be early warning signals for the latter—a hypothesis that could be tested using future data, such as recent cross-Strait diplomatic events.
Early Warning for the Next Taiwan Strait Crisis
We test the algorithm’s performance against five sets of recent Taiwan Strait events:
- Then-US House Speaker Nancy Pelosi’s August 2022 visit to Taiwan and China’s subsequent military escalations
- Then-Taiwanese President Tsai Ing-wen’s April 2023 visit to the US and meeting with then-US House Speaker Kevin McCarthy, as well as China’s response
- Then-Taiwanese Vice President Lai Ching-te’s August 2023 visit to the US and China’s response
- Lai's inauguration as the Taiwanese president
- Lai’s visit to the US in November 2024 for the first time as Taiwanese president, while China’s response was relatively muted
Diplomatic activities of the Taiwanese government, especially those involving its US counterpart, have traditionally been a thorn in China’s side, but the resulting level of cross-Strait tensions varies substantially. Pelosi’s visit to Taiwan triggered an escalatory spiral among China, Taiwan, and the US, significant enough for some observers to consider it another Taiwan Strait Crisis since the 1995 episode.[14] In contrast, the most recent US visit by Lai did not generate much traction in the news cycle.
The right column of plots in figure 1 validates these impressions but more quantitatively. The events surrounding Pelosi’s Taiwan visit exhibited the expected behavior across the four indices, with the most pronounced changes occurring shortly before her arrival in Taiwan. This is followed by Lai’s inauguration as the Taiwanese president, which registered a significant dip in China’s attitude toward Taiwan and a sizeable increase in the prospect of military activities across the Taiwan Strait. In contrast, other events listed above received relatively muted movements across the four indices.
Granted, events serious enough to be considered a Taiwan Strait crisis are few and far between, but the patterns captured by PCI-Tensions seem to have the potential to be early warnings for the next crisis, which could be the prelude to a Chinese invasion of Taiwan—if and when it occurs.
Concluding Remarks
We see two extensions of the algorithm that could potentially improve its performance in predicting future Taiwan Strait crises. First, there were other crises before 1995, such as the ones in 1954–1955 and 1958. Including these precedents would allow the algorithm to have more training data, although the potential differences in the text data due to the long time apart would need to be accounted for.
Second, to the extent that a crisis in the Taiwan Strait would likely involve not only China and Taiwan but also the US and its Indo-Pacific allies, media data from these other countries could potentially contain useful information for the model. However, because these other countries are liberal democracies, their media data must be treated differently from that of an authoritarian mouthpiece like People’s Daily. We relegate these interesting yet challenging extensions to future investigations.
The dominoes triggered by a Chinese invasion of Taiwan would have a catastrophic impact on the world.[15] Predicting the likelihood and timing of such an event is exceptionally difficult. Fortunately, propaganda analysis, which relies solely on public information, along with modern AI technologies, could help us take a step closer to that goal.
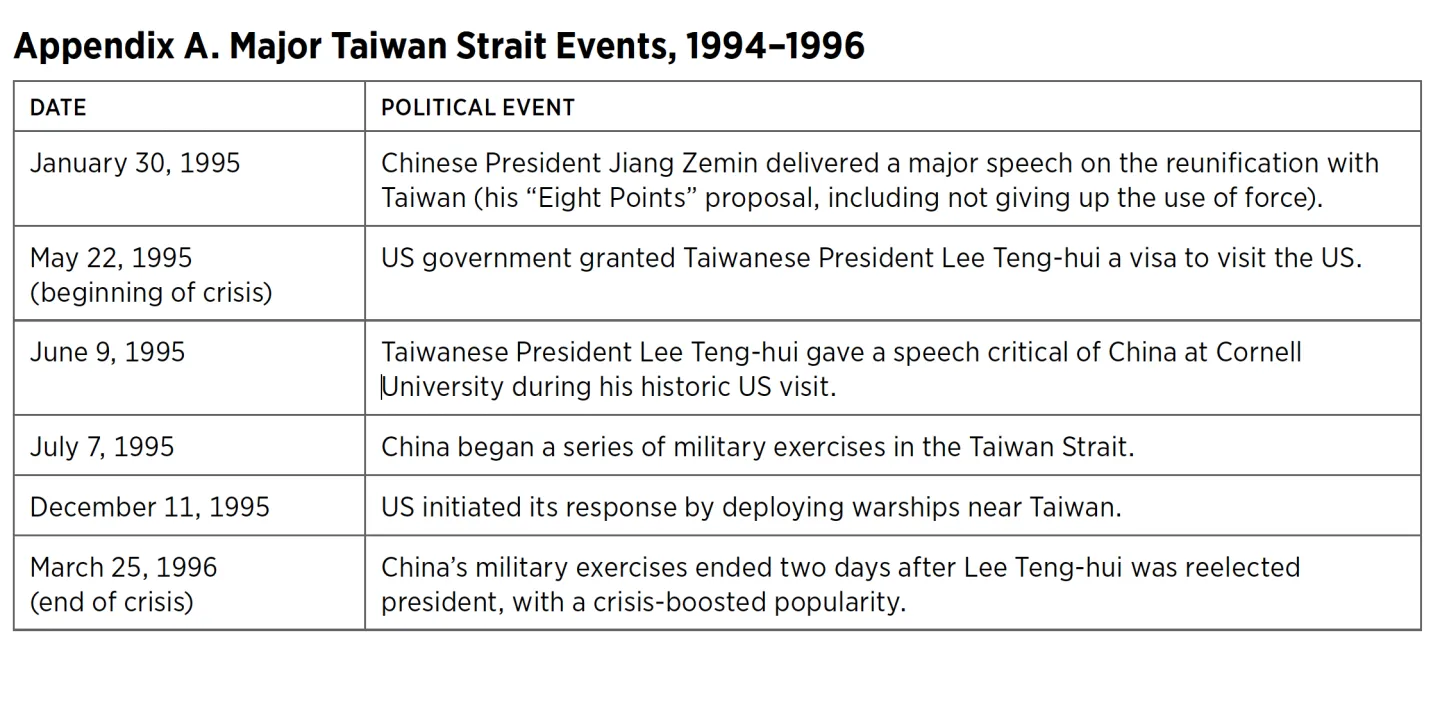
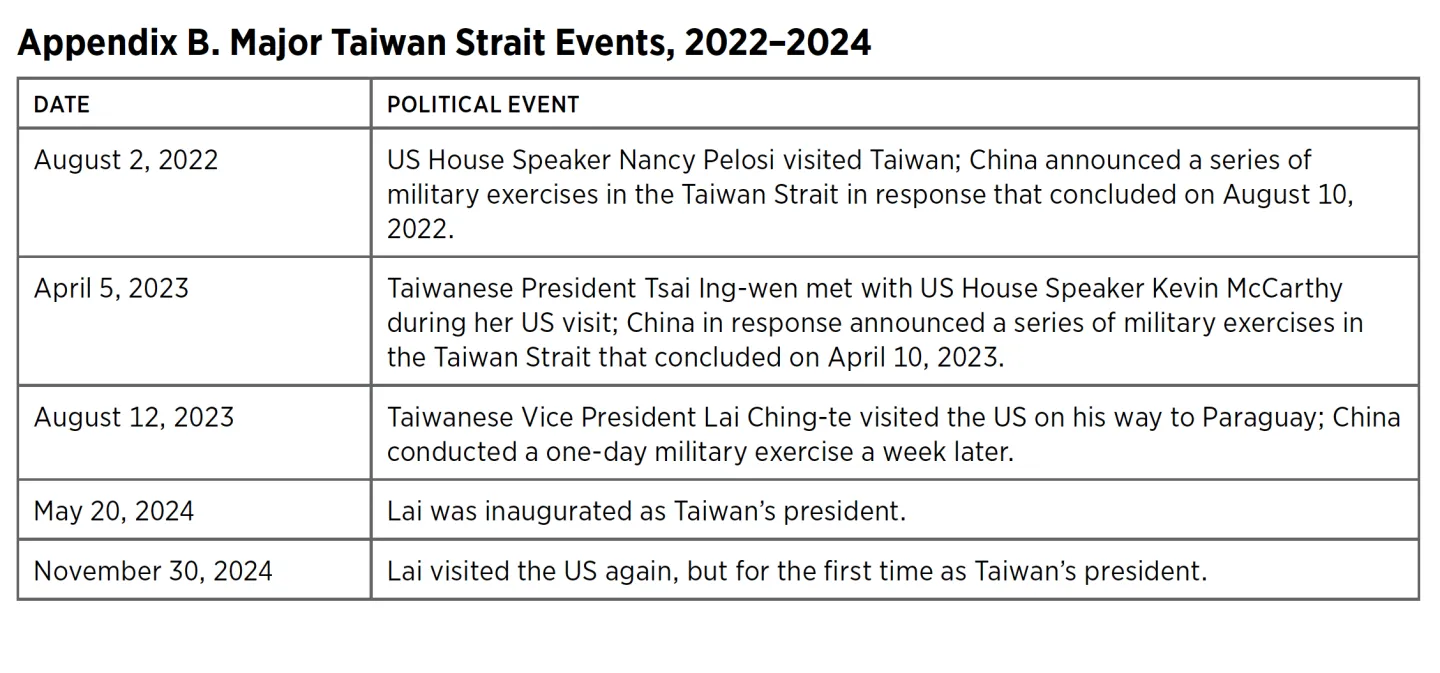
About the Authors
Kaiwei Hsu is a senior economist at Bates White Economic Consulting, and his work focuses on antitrust economics. Hsu has a PhD in economics from the University of Chicago. He is also an external contributor to the open-source Policy Change Index project (policychangeindex.org).
Weifeng Zhong is an affiliated scholar at the Mercatus Center at George Mason University and a senior advisor to the Office for Fiscal and Regulatory Analysis at the America First Policy Institute. Zhong has a PhD in managerial economics and strategy from Northwestern University. His work bridges the fields of machine learning, artificial intelligence, and public policy studies. He is also a core maintainer of the open-source Policy Change Index project (policychangeindex.org).
Notes
This project is a collaboration between the Policy Change Index Project and Gear Factory, a company specialized in deploying artificial intelligence solutions. We thank Jack Zhang for his helpful comments on dating geopolitical events. We also thank Kai-Chieh (KJ) Hsu for the helpful comments on the interpretation of LLM results. The views expressed here are solely the authors’ and do not represent the views of Bates White, the Mercatus Center, the America First Policy Institute, or their other employees.
[1] Alex Willemyns, “When Might China Invade Taiwan? Depends Who You Ask,” Radio Free Asia, January 30, 2023.
[2] Bonny Lin et al., Surveying the Experts: US and Taiwan Views on China’s Approach to Taiwan (Center for Strategic and International Studies, January 22, 2024).
[3] Julian TszKin Chan and Weifeng Zhong, “Reading China: Predicting Policy Change with Machine Learning” (AEI Economics Working Paper No. 2018-11, American Enterprise Institute, 2019).
[4]Fred S. Siebert, Theodore Peterson and Wilbur Schramm, Four Theories of the Press: The Authoritarian, Libertarian, Social Responsibility, and Soviet Communist Concepts of What the Press Should Be and Do (University of Illinois Press, 1984).
[5] Eisenhower, Crusade in Europe, p. 260.
[6] Alexander L. George, Propaganda Analysis: A Study of Inferences Made from Nazi Propaganda in World War II (Row, Peterson and Co., 1959).
[7] Weifeng Zhong, “How the Policy Change Index Can Help Us Understand China’s Next Moves” (Mercatus Policy Spotlight, Mercatus Center at George Mason University, March 19, 2024.
[8] This section describes the algorithm at an abstract level. For details, please see the PCI website at https://policychangeindex.org/ and the open-source code repository at https://github.com/PCI-ORG/PCI-Tensions.
[9] We follow the International Crisis Behavior (ICB) Project, (see https://cidcm.umd.edu/research/international-crisis-behavior-project), in its determination of start and end dates of the 1995 Taiwan Strait Crisis. For more recent events not yet codified by the ICB, we date the events by following the ICB approach as closely as possible.
[10] At the time of this writing, we use GPT-4o mini, a versatile and cost-efficient model developed by OpenAI, but this choice can easily be modified in future and other applications.
[11] Specifically, for each topic, we first estimate a rating using the LLM for each article and calculate an aggregated weekly rating by summing ratings across all articles in a week. To filter out random fluctuations over time, we then calculate the four-week simple moving average using the ratings over the last four weeks.
[12] This is the only time the test period data are used in the development of the algorithm.
[13] By repeating steps 3 and 4, we have experimented with over a dozen other prompts and topics, and the results presented here are robust against those variations.
[14] China Power Team, "Series: The Fourth Taiwan Strait Crisis," China Power, April 26, 2023. Updated November 5, 2024, accessed March 8, 2025, https://chinapower.csis.org/series-fourth-taiwan-strait-crisis/.