Artificial intelligence, and the policy response to it, is at an inflection point. And with hundreds of definitions of AI and a sudden explosion in AI progress, now more than ever policymakers must acquire a deeper understanding of the technology to navigate its impact on society.
Updated September 2024
This part of the AI Policy Guide discusses the paradigm shift in AI and the need for policymakers across federal agencies to have a deeper understanding of the technology.
In 2022, the release of ChatGPT took both the public and policymakers by surprise. Accessible, conversationally fluid, and helpful across a breadth of domains, artificial intelligence (AI) finally started to distantly resemble sci-fi promises. We are clearly at an AI inflection point, but what has changed? First is scale. Near the turn of the current decade, three forces converged to enable large, massively intelligent models: the flexibility of new, highly scalable algorithmic techniques, big data amassed at scale to provide necessary knowledge, and the wickedly fast chips to handle AI’s highly intensive data and computation. Second, and perhaps more important, is breadth. In 2021, Stanford University’s Human-Centered Artificial Intelligence Institute wrote about a broad, profound AI “paradigm shift” arising from these convergent technologies. The result of AI’s new scale was a new class of so-called foundation models—large-scale systems trained on broad sets of data that can be easily adapted or fine-tuned to a wide range of downstream tasks. Armed with computational heft and flexibility, these models offer many of the tools needed for AI to step beyond a mere curiosity.
Even with a measure of skepticism toward the AI hype, recent developments illustrate that the impact of AI is simultaneously emerging across a multitude of domains:
AlphaFold predicts the structure of nearly every known protein—a critical new tool in biological and medical research.
Midjourney’s art generator produced near-human-quality works.
AlphaTensor discovered a more efficient approach to matrix multiplication than previously known, and this could soon speed up a wide range of applications.
Insilico Medicine’s proprietary systems created a potential treatment for idiopathic pulmonary fibrosis, the first AI drug to enter FDA phase II human trials.
MūtCompute discovered an enzyme that breaks down polyethylene terephthalate, a common plastic that represents 12 percent of global waste.
GraphCast provides 10-day weather predictions with state-of-the-art accuracy in under a minute.
OpenAI’s ChatGPT produces logically complete text and image responses to user queries.
Note the range of fields. AI is being applied everywhere—from the arts and linguistics to chemistry and pure mathematics. It is flexible. The tools that make AI possible represent a new class of general-purpose technologies, innovations that “[have] the potential to affect the entire economic system.” Just as previous general-purpose technologies such as electricity transformed society, AI systems are changing many domains—from science to entertainment, from education to health, from national defense to the financial system—and could even radically transform them.
Some critics claim that these advances in AI are skin deep, mere “stochastic parrots” that randomly rearrange and regurgitate data. They may look effective, critics argue, but AI lacks any true understanding, common sense, or ability to explain their decisions. There is ample room for debating the nature of true intelligence, and critics will err dramatically if they dismiss AI outright as unimportant. The future of AI is unclear, but the increasing breadth and scale of AI applications demands attention from an increasing breadth of decision makers.
Yet policymakers are often not keeping pace.
Policy decisions about AI made today may hold long-term importance for this technology’s future. While knowledge is no “good government cure-all,” it is a necessary first step for thoughtful decision-making. In this work, we hope to impart a basic understanding of AI design, application, and policy challenges to inform policy-minded readers.
The Tip of the AI Policy Iceberg
What do we lose without a diversity of experts engaging with AI in depth?
“However brilliant computer engineers may be when facing down technological challenges, they rarely have real insight into what’s happening outside the digital bubble.”
—Jacob Helberg, former Google news policy lead; commissioner, US China Commission
In summer 2022, AI image generation seemed to appear out of nowhere. With the release of DALL·E mini, an open-source approximation of OpenAI’s DALL·E 2 art generator, AI art was suddenly accessible to everyone. Delighted by the often strange yet sometimes human-quality works, consumers flocked to the application and flooded social media with bizarre AI creations. Powerful enough to wow yet amusingly inaccurate, DALL·E mini introduced many to a glimpse of the possibilities of image generation while comforting others with the understanding that generative AI was still out of immediate reach. Yet, in just a matter of weeks, things changed. OpenAI broadened access to the full version of DALL·E 2, Midjourney generated covers for The Economist, and Stability AI released the powerful Stable Diffusion—in just one summer these wonky generators suddenly proved capable. Since then, generative tech has matured and broadened, perfecting images while making headway in video, audio, and video games.
This sudden burst of innovation caught policy officials off guard. In a matter of weeks, copyright, intellectual property (IP), and other media-relevant officials had to shift gears toward confronting an unexpected slew of novel AI-based issues far from consideration just months earlier. One notable controversy: artistic rights. To develop these systems, engineers scraped volumes of preexisting human-crafted works from galleries across the web, leaning on those data to hone their model’s image-crafting abilities. Often, this process was undertaken without artistic consent. As a result, prominent digital artists found that this software could produce near-perfect renditions of their works, allowing anyone to appropriate signature styles. This situation raised challenging questions of usage rights, privacy, personal autonomy, and copyright infringement. Since 2022, the controversy has led to growing artistic agitation. Online, creators have attempted to disable image generators through intentionally corrupted data. In industry, the 2023 Hollywood strikes struck back at potential corporate application. In government, policy remains under deep IP uncertainty.
At the time, many affected artists viewed this situation as potentially existential. For those at the top levels of AI policy when DALL·E first arrived, however, it was off-radar. Interviewed on the effect of image generators in late 2022, one member of the National Artificial Intelligence Research Resource Task Force, the nation’s top AI policy advisory panel, had not even heard of the issue. It’s likely the broader task force was also in the dark.
The reason? AI had been treated as a technical specialty. Because the task force was composed almost exclusively of computer scientists, one is hardly surprised that it was not thinking about artistic rights questions. Had media policy officials recognized AI’s coming general-purpose breadth, perhaps those in the arts would have been engaged in policy and had their voices heard in the design of potential solutions. A second challenge is prediction. Breadth of expertise cannot sacrifice depth of technical knowledge. Only by understanding the technical progress of generative AI—how data are scraped and used to train AI and what type of data are needed—could those concerned about artistic rights have perhaps predicted this issue and have begun to consider appropriate action. Many generators are now open sourced, meaning their code is no longer controlled by a single entity, and affected artists may have little recourse. Solving this issue would have required forward thinking, knowledgeable officials aware of technical trends, and dedicated AI policy work in media and artistic rights policy. In AI policy, such specificity is often missing.
AI Touches All Federal Departments
Artificial intelligence (AI) has a broad effect. One can see how it is actively affecting policy in each federal department and across disparate policy areas:
The Importance of Deeper Understanding
The sudden explosion in AI progress demands a new class of policymakers who not only understand AI, but also understand it in depth.
To manage impactful technology, we must broadly equip officials. The National Security Commission on Artificial Intelligence recently wrote that “AI … promise[s] to be the most powerful tools in generations for expanding knowledge, increasing prosperity, and enriching the human experience.” All policy areas will be touched and even transformed by AI. The sudden explosion in AI progress demands a new class of policymakers who not only understand AI, but also understand it in depth. Just as all policy experts need a working knowledge of economics, all will need a working understanding of AI.
Traditionally, those who have engaged with AI outside computer science have done so only at a basic level, a so-called Level 1 understanding. They can engage with the concept, and perhaps entertain abstract effects, but cannot dig into problems or imagine specific solutions. AI is maturing, and policymakers should go deeper. The goal should be a Level 2 understanding, in which policymakers understand conceptually how AI works and the array of core concepts and technologies on which it is built. Although they might not be able to code a neural network, they know how one functions. Although they have not studied electrical engineering, they understand the AI chip deck.
With a Level 2 understanding, this new class of policymakers can meet engineers halfway. More specifically, they will have the confidence to ask the right questions, the ability to understand engineers’ explanations, and, crucially, the capability to question technical experts. This level of understanding brings AI down to earth, allowing policymakers to see the breadth of AI’s effect and the many technical tools on which it is built.
How to Use This Work
The goal of this work is to equip a diversity of policymakers with the core concepts needed to acquire a degree of understanding. While a Level 2 understanding is the goal, in each section we offer two levels of depth to support readers who want only a basic understanding and those seeking greater depth.
Note that AI is enabled not by one technology but rather by a diverse “constellation of technologies.” AI comes in many forms and uses a range of concepts and devices. To understand and solve diverse AI issues, readers must grasp the AI space. Primarily, this work seeks to explain how AI works through illustration. Along the way, it equips readers with key terms, fundamental concepts, and core technologies in a toolbox of knowledge that can be supplemented with application-specific expertise.
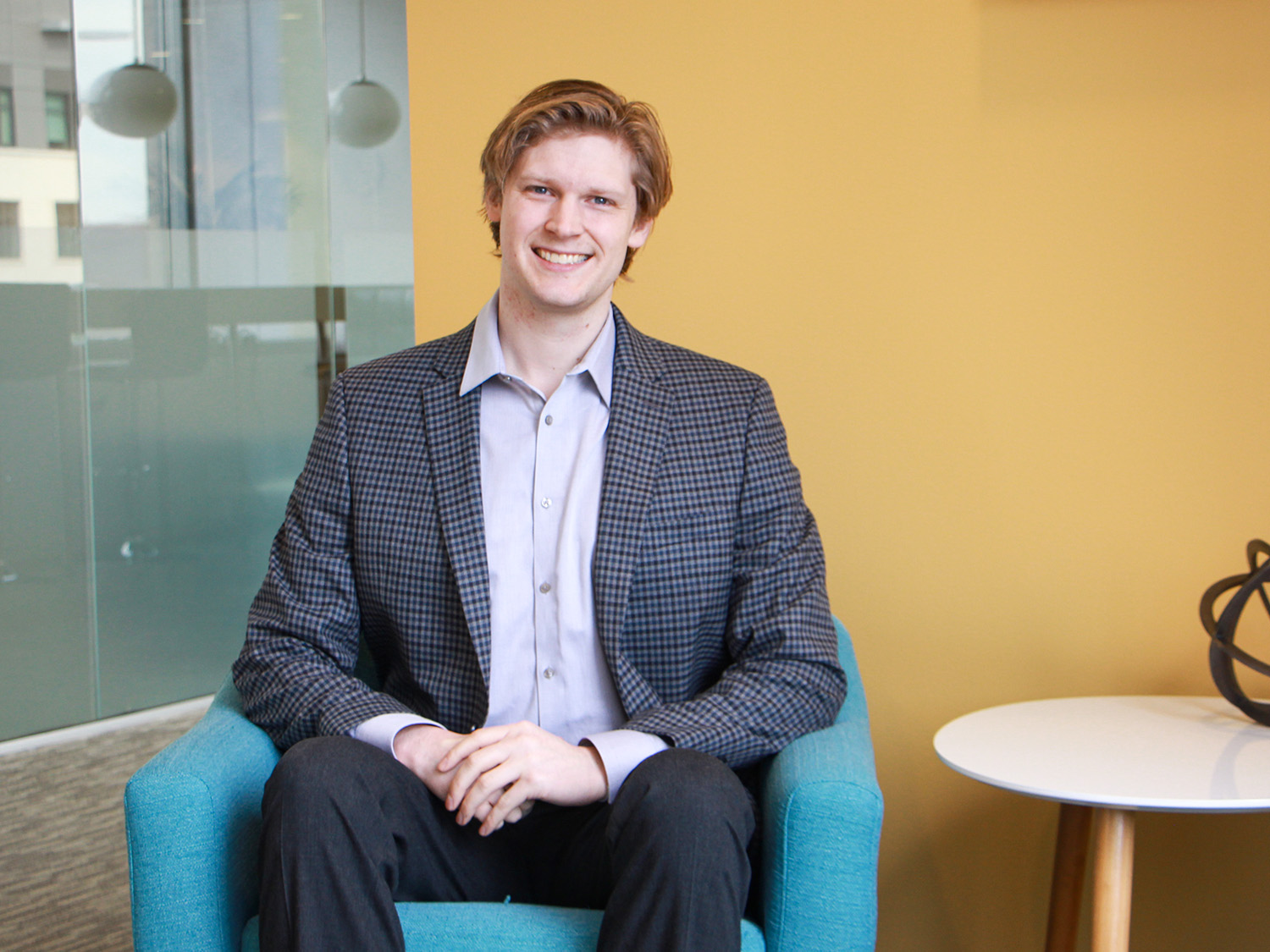