What Is AI?
AI is difficult to conceptualize and define. How can we define artificial intelligence when we don't understand what regular intelligence is either? While Congress simplified these questions by hard coding an AI definition into law, this definition must be rooted in a practical understanding of this often hazy concept.
Updated September 2024
This part of the AI Policy Guide provides an overview of what characterizes artificial intelligence, including an explanation of the legal definition codified by Congress, the core input technologies of AI, and a high-level overview of how AI often works.
Artificial intelligence (AI) is characterized by the following:
Normatively, AI can be thought of as a goal—the goal of using human-designed systems to build something intelligent, often resembling the human mind. Descriptively, AI is commonly considered a technology, a catch-all for the many technologies and designs that make AI possible.
AI systems generally aim to automate intellectual tasks normally performed by humans.
Technologies such as machine learning are used to create AI systems.
Most AI systems are best conceived as advanced inference—or prediction—engines. These inferences are used to produce analysis, inform decisions, and take automated actions.
AI is the result of a triad of essential inputs: software (algorithms), hardware (microchips), and data.
The core advantages of AI systems are advanced automation, analytical speed, and greater scale of action.
While AI systems have traditionally been geared toward narrow applications, more general-purpose systems are emerging. Despite widespread attention on these general-purpose systems, the bulk of systems in use are designed for discrete, narrow-use cases.
An algorithm is simply a logical sequence of steps needed to perform a task. In computer science, algorithms are written in code.
Machine learning algorithms are often trained with data stored in a databank or collected in real time.
AI Basics
This section provides a basic, Level 1 understanding of AI, including AI’s benefits, system flexibility, and the way AI works.
“A fundamental problem in artificial intelligence is that nobody really knows what intelligence is.”
There is no one accepted definition of AI; there are, in fact, hundreds. For policy experts, Congress thankfully simplified definitional selection by hard coding an AI definition into law through the National Artificial Intelligence Initiative Act of 2020. Legally, AI is defined as follows:
A machine-based system that can, for a given set of human-defined objectives, make predictions, recommendations or decisions influencing real or virtual environments. Artificial intelligence systems use machine and human-based inputs to—(A) perceive real and virtual environments; (B) abstract such perceptions into models through analysis in an automated manner; and (C) use model inference to formulate options for information or action.
This definition is wordy, but a few core concepts stand out.
Intelligence. First, note that the legal definition just mentioned does not explain the goal of AI technology. The reason: the goal is in the name. As observed earlier, artificial intelligence itself is a goal enabled by a set of ever-changing technologies (for example, machine learning). The bounds and aims of this goal are naturally murky because there is little consensus on what constitutes “intelligence.” Some believe serious research should downplay mimicking intelligence, and specifically human intelligence, as the end goal, with emphasis alternatively placed on advanced task automation, data analysis, and other goals to set expectations. That said, several organizations today, such as OpenAI, are explicitly seeking to produce generally intelligent, human-level (or beyond) systems. Mimicking human intelligence was the goal of AI’s founders, and most watershed moments in AI history such as AlphaGo’s mastery of the game Go involve outmatching specifically human intelligence. Although defining intelligence is murky, there is no question that many AI engineers (for better or for worse) will keep some notion of human intelligence as their ultimate goal.
Readers should take this approach with a grain of salt. Focusing too intently on efforts to mimic human thought can distort our understanding of what an AI system is or represents. It also distracts from progress in the many systems that aren’t trying to achieve human or general intelligence. Today, most AI systems are narrowly scoped, seeking complex task automation. Facial recognition systems, for instance, are not trying to create human intelligence; they are trying to automate human identification.
Regardless of the aim or application, modern AI systems are united by a general attempt to “automate intellectual tasks normally performed by humans,” an effort naturally shaped by the application at hand and the personal views of its engineers.
Inference. A second highlight from this definition is that machine-based systems “make predictions, recommendations or decisions.” In the field, this is called inference. Inference is at the core of all systems, and the goal of AI systems can be generalized as the goal of making good inferences. When one asks the Alexa voice assistant to play a song, it infers a song title on the basis of the sound of the words, triggering instructions that compare that inferred title against other titles in its database. It then plays the most likely match. Similarly,
Identifying and labeling the contents of a picture means inferring the correct match between the input image and potential labels.
Autonomously operating a car requires thousands of near-instant inferences about which actions to take in the near future—that is, predictions based on the position of the vehicle and surrounding objects and other information.
When these inferences trigger machine action (such as playing a song or steering a car), AI achieves the goal of automation.
The AI Triad. A third highlight is the phrase “machine-based systems.” AI scholar Ben Buchanan explains that “machine learning systems use computing power to execute algorithms that learn from data.” This is the AI triad: algorithms, data, and microchips—the core input technologies that together enable AI. An essential theme of this introduction is that each of these technologies is equally necessary and interdependent. Understanding this interdependence is key to designing AI policy.
Benefits of AI
Before diving into how AI works, one must form an idea of what AI systems offer:
- Automation. AI can automate new types of tasks that previously required human input. Before AI, automation was reserved for the consistent, predictable, and repetitive. AI expands automation into “fuzzy” tasks that deal with complex problems and uncertainty. With AI, automation can extend to imprecise tasks including image recognition, speech translation, and writing.
- Speed. AI can resolve complex problems nearly instantly. Driverless cars face no cognitive lag when responding to hazards, and ChatGPT faces no analysis paralysis when writing. Decisive, near-instant decisions provide an advantage over human decisions, which can lag as a result of indecision, stress, and other factors. In other cases, speed can also be a hazard of its own. An extreme example lies in military systems that once granted autonomy over target engagement, allowing action before a human commander authorizes engagement.
- Scale. AI can effectively perform certain tasks better than an army of humans hired for that purpose. For instance, streaming can simultaneously address the individual preferences of millions of music listeners or TV viewers, drug discovery systems can analyze millions of compounds, and ChatGPT can search and connect millions of disparate ideas.
System Flexibility
Today, all AI systems can be categorized as artificial narrow intelligence, designed to perform a specific, limited function set. (Narrow AI is often alternatively referred to as “brittle.”) These AI systems can perform one or a few tasks with high quality but cannot perform tasks outside their discrete training.
AI applications range from single-purpose systems, such as OpenAI’s DALL·E image generator, to more complex systems such as driverless cars or even ChatGPT. Even within these narrow domains, AI can still suffer inflexibility. Generalization refers to a system’s ability to “adapt properly to new, previously unseen data”—that is, it can flexibly adapt to novel scenarios it hasn’t been explicitly trained to handle. The more a system can generalize and deal with the unexpected corner cases in its domain, the higher its quality. Imagine a driverless car that is highly accurate but only in average, fair-weather road conditions. This car would perform perfectly in the majority of cases, yet when it meets a rare and unexpected situation—say, a tornado—it may not know the best course of action to protect the driver.
Although today’s AI systems are narrow in scope, efforts are under way to develop so-called artificial general intelligence (AGI), which has “the ability to achieve a variety of goals, and carry out a variety of tasks, in a variety of different contexts and environments.” This category represents the science fiction vision that many readers hold of AI. Note that generality does not imply balanced quality across capabilities. Just as a lion might excel at hunting and a human at mathematical reasoning, it is possible for AGI systems to perform tasks at varying levels of proficiency. Also note that AGI does not imply humanlike AI; AGI can be as advanced as humans without necessarily mimicking our cognition. A chess-playing AI, for instance, might win by mere exhaustive calculation of every combination of possible moves. Contrast this thought process with the strategic reasoning of human cognition. AGI also does not mean superintelligence—that is, an AI system that is smarter than humans in almost every domain. These variations on advanced AI systems do not yet exist, though increasing R&D has been devoted to their development.
Policymakers should take these concepts seriously even if they consider true AGI far off or impossible. Even an AI that can convincingly mimic AGI or superintelligence ought to be a matter of policy concern.
How AI Works: Prerequisites
The following sections discuss the various elements of the AI triad and the way AI works. First, several basic terms and concepts are as follows:
Algorithm. “A logical sequence of steps to solve a problem or accomplish a task.” Although this term sounds to some like technical jargon, algorithms are everywhere. For instance, Grandma’s pot roast recipe is a type of algorithm: a list of steps that, if followed, can produce the delicious Sunday dinner. In computer science, this term is more specific, referring to the list of instructions, or code, that a computer follows. The essence is still the same; the computer follows lines of code to perform its tasks just like one might follow a recipe. The term is often used interchangeably with computer program and software.
Although this guide defines algorithm in its most general sense, in the context of AI, “algorithm” is often used as shorthand to refer more specifically to machine learning algorithms,the processes that a computer follows to create artificially intelligent software.
- Model. Unlike the more general term “algorithm,” the model is the software configuration that, once fed input data, can produce output inferences, predictions, and decisions. The model is the end result, which is the inference software created from the iterative refinement of machine learning or engineering. When one trains an AI system, one is training the model; when one runs an AI system, one is running the model.
- Machine learning. Most AI systems today are the result of a process called machine learning. Machine learning is a method for iteratively refining the process a model uses to form inferences by feeding the model stored or real-time data. This learning process is called training and is a necessary step to build artificially intelligent systems. In the “Algorithms” section, this process is explained in greater detail.
AI in Detail
This section provides a more advanced, Level 2 understanding of AI, including assessing quality and accuracy and understanding benchmarks.
In additiona to understanding what AI is and how it works, many policymakers must know how to assess it. Unfortunately, there is no one performance metric for AI models, and the measurement criteria used are highly specific to each application and are constantly changing. This study offers a starting point, describing several common metrics and the way to approach these figures with a critical eye.
Accuracy Assessments
A natural starting point for quality assessment is accuracy, which measures how a system’s inferences and actions match expectations. Accuracy is broadly useful, understandable, and often sufficient. Note, however, that perfect accuracy will rarely be possible. When deploying AI applications, engineers must actively decide on an acceptable rate of failure (a choice based on their own reasoning), application requirements, and perhaps regulatory prescriptions. Alexa, for instance, answers incorrectly around 20 percent of the time. In Amazon’s estimation, this rate of failure is acceptable. This estimation illustrates that accuracy need not be perfect when the stakes are low.
Contrast this example with safety modules in a driverless car. In this case, many argue that, given the danger, the acceptable level of accuracy must be higher. Safety still must balance practical considerations. Projections show that deploying a driverless car that is only 10 percent safer than one with human drivers could still save many lives; perhaps a seemingly high rate of failure might be acceptable if it still minimizes comparative risk. Other AI benefits must also be weighed against accuracy. Conceivably, driverless cars could efficiently clear traffic in the presence of ambulances, potentially saving lives. Perhaps such a benefit would justify a lower rate of overall accuracy.
Accuracy Is Not Everything
Accuracy, although an important metric, cannot fully assess system quality in all cases. For instance, if a deadly virus appears only once in a sample of 100 patients, a disease-spotting AI coded to always predict a negative result would still be 99 percent accurate. Although highly accurate, this system would fail its basic purpose, and the sick would go untreated. For policymakers, a critical eye is needed to ensure that the numbers provide proper nuance.
To gain a better sense of the quality of a system, one may need additional evaluation metrics. It is important to emphasize that any metric used to evaluate AI carries tradeoffs. As an illustration, there is often a tradeoff between measuring false positives and false negatives. Choosing which to prioritize in evaluation depends on context and system goals.
Returning to the disease-detecting AI example, suppose one is doing aid work for the United States Agency for International Development. The chief concern is treating disease, and there is no cost to treating a healthy patient. In this case, one might prioritize minimizing false negatives so as to ensure that those with the disease get treatment. Also, one might measure quality using recall, a metric that states the percentage of the model’s negative results that are true negatives. This metric would allow one to see the likelihood of a false negative, and if that probability is low, the model is effective for our purposes.
Now imagine the reverse: suppose one is an official at the Centers for Disease Control and Prevention, and the chief concern is correctly analyzing disease transmission. In this scenario, perhaps one would want to minimize false positives by measuring with precision, a metric that evaluates how many positive results of the system are indeed positives. If precision is high, then one can be certain that one is correctly identifying positive results and can better track transmission.
If one finds both false positives and false negatives undesirable, perhaps one wants a model that minimizes both. In this case, one would try to maximize the F1 score, assessing how well the model minimizes both false negatives and false positives.
These example metrics are widely used to assess AI that seeks to classify data; however, that is only one aspect of evaluation, and it is not necessarily ideal for all applications. Consider how one might assess the quality of art-generation software. This task is naturally fuzzy and, in many cases, depends on the priorities or tastes of individuals; this is not something that can be easily captured in statistical metrics. A 2019 study found that for generative adversarial networks (GANs)—an AI model that can serve as an AI art generator—there were at least 29 different evaluation metrics that could be used to assess the overall quality of these systems in different contexts. AI evaluation metrics, like AI itself, are meaningless without application.
Benchmarks
Although evaluation metrics can usefully describe an individual model’s effectiveness, they are not suited for comparing models or tracking progress toward certain goals. As such, AI researchers have adopted a variety of benchmarks, common datasets paired with evaluation metrics to allow model comparison and results tracking and determine state-of-the-art performance on a specific goal or task. These benchmarks are often tailored to specific tasks, goals, and complexities. For instance, ImageNet benchmarks image detection and classification, while HellaSwag benchmarks a chatbot’s commonsense reasoning.
Although useful for tracking improvements in AI systems and the state of the art, these benchmarks can be limited in their descriptive abilities. Researchers have noted that while benchmarks are often seen as describing general AI abilities, what they actually represent is more limited in scope, measuring only a system’s ability at the tightly constrained benchmarking task. Even if an AI system is able to accurately identify most images in ImageNet’s database, that action does not necessarily mean those abilities will translate to real-time, real-world image recognition. The complexity and noise of real-world analysis can be a far cry from the limited frame of benchmarking tests. Further, it has been noted that benchmarks often fail to test necessary characteristics such as a model’s resistance to adversarial attacks, bias, and causal reasoning. Benchmarks are constantly being replaced, supplemented, or updated as these limitations are discovered.
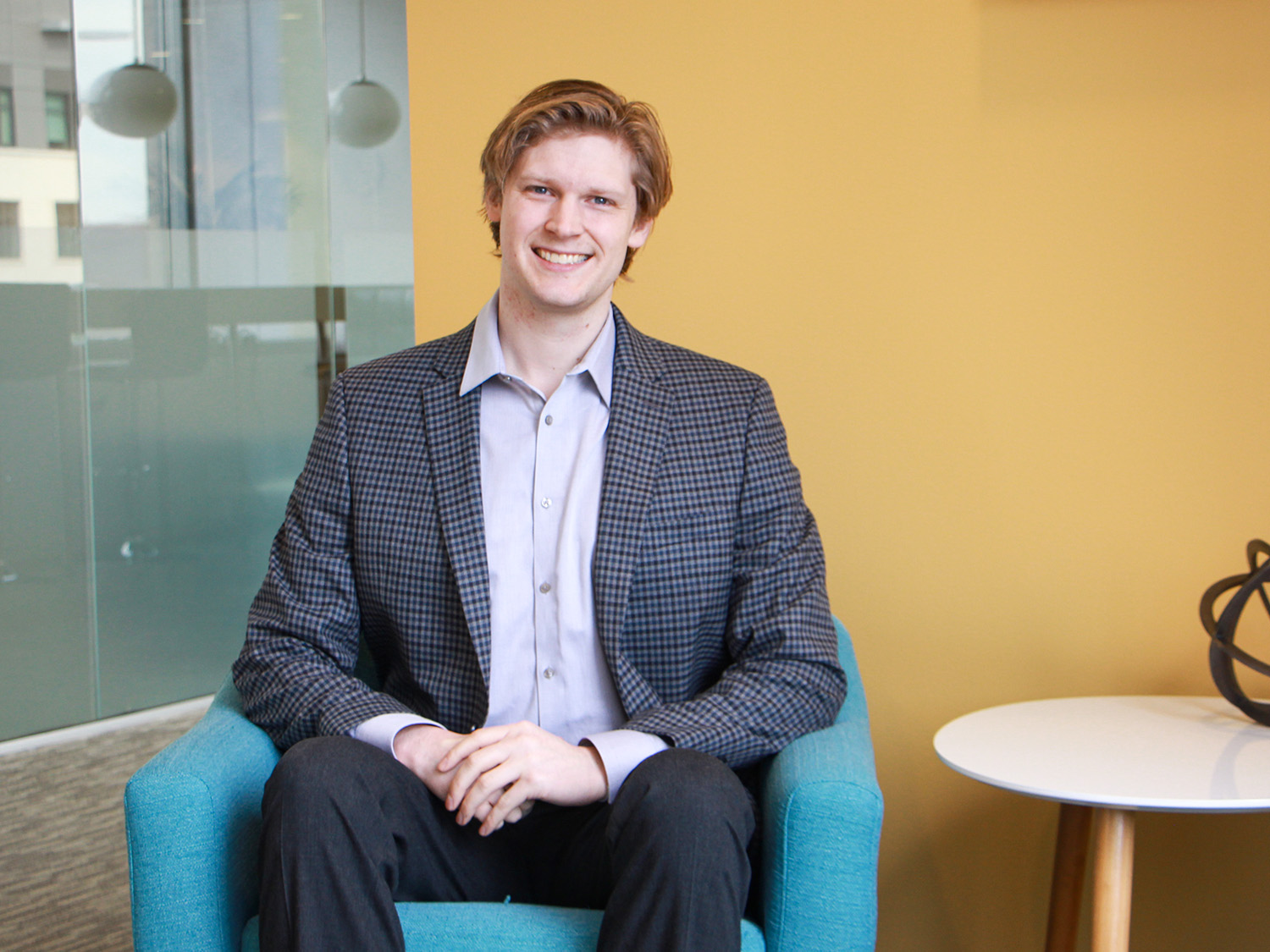