AI Policy Challenges
From chip development to supply chain security, policymakers must navigate complex challenges to ensure AI has a net benefit for society. AI policy is still in its infancy, and effective governance requires policymakers to level up their understanding of the underlying technology because of the potential breadth and depth of its impact.
Updated September 2024
This part of the AI Policy Guide covers several areas of active policy concern, including chip development, supply chain security, and algorithm development. It highlights the questions of government R&D support, supply chain security, externalities, talent, and bias.
Before digging into the technology that makes AI possible, we must first establish what artificial intelligence (AI) policy looks like today and what issues are at stake. Currently, there is limited artificial intelligence–specific law. Only a handful of federal laws relate directly to AI, and those that do, such as the National Artificial Intelligence Initiative Act of 2020, cover basic study and coordination rather than explicit regulation.
There is comparatively more AI-specific policy and executive action, though this too is in introductory stages. The National Institute of Standards and Technology’s widely used AI Risk Management framework provides optional processes and considerations for organizations looking to responsibly and safely deploy and develop AI systems. The 2022 Blueprint for an AI Bill of Rights lays out a list of principles officials believe should guide AI application and policy. More substantially, 2023’s Executive Order on the Safe, Secure, and Trustworthy Development and Use of Artificial Intelligence acts as the guiding document of AI strategy in the United States. This lengthy list of requirements includes limits on government use of AI, and it requires chief AI officers in most agencies, AI talent development initiatives, and technology reporting for frontier AI labs. Beyond these specific actions are introductory requests to a range of agencies to consider research, investigation, or even actions on critical infrastructure risks, civil rights, market competition, intellectual property, AI bias, and consumer protection, among other steps.
Such executive actions depend on a range of preexisting general-purpose statutes that apply to and can regulate all technologies, AI included. For instance, 2023’s AI executive order used the Defense Production Act’s industrial base assessment powers as the basis for its new technical reporting requirements for frontier AI labs. Likewise, the Federal Communications Commission ruled that, under the Telephone Consumer Protection Act, AI-generated voices in robocalls are illegal. Other general-purpose statutes regulating domains include consumer safety, transportation, intellectual property, healthcare, national defense, the justice system, and discrimination (among others) that likely also will apply to specific AI systems in certain circumstances.
At the state and local levels, policy is varied and often more application specific. In many states, actions have been targeted at limited-scope and well-publicized AI applications and issues. These applications include “deep fakes,” AI-generated election materials, autonomous vehicles, and AI-assisted hiring. Following ChatGPT’s release, some states have considered various forms of broad, comprehensive AI regulation, though no such bill has passed. In all cases, there is clearly a desire to act (perhaps regulate) and manage certain AI risks. What that may look like and how state legislation interacts with federal legislation remain to be seen.
The design of AI law and policy is and will be a complex task because of the importance and wide reach of this technology. The following sections offer a few questions that policymakers should consider when designing AI policy.
Critical Questions for Policymakers
Policymakers face many important decisions in the areas of research, development, and manufacturing; inputs and resources; quality control; externalities; and security and safety. This section discusses each in turn.
Note that related to each issue is a broader question of implementation and governance. Because AI broadly impacts society, policymakers must consider how to structure regulatory and policy governance. They should consider these high-level questions:
Should there be a dedicated “AI agency,” or should policy and regulation be devolved to domain-specific agencies? What problems would a potential new agency solve? What would its jurisdiction be?
What gaps and overlaps in law and policy might hinder clear, effective policy? How can we identify those gaps and overlaps?
Research, Development, and Manufacturing
Chip development. Historically, the US government has sponsored and supported AI chip development. The recent Chips and Science Act illustrates the support of the semiconductor industry by policymakers of both parties. This legislation follows a long history of public engagement with this sector. While the issue has enjoyed congressional support, the utility of AI industrial policy has been the subject of considerable debate, including the following questions:
Is there certain fundamental AI chip research that might not exist without government support?
Does government support and subsidization risk crowding out or privileging certain innovations and alternative designs?
How can policy play a role in ensuring that US industry competes with China’s considerable state-led AI investments?
Computer science and algorithmic research. Algorithm and computer science research has long been intertwined with public research support and policy. Early neural networks, for instance, were first introduced by the Office of Naval Research. The Defense Advanced Research Projects Agency’s Grand Challenge, a military-sponsored desert race, sought to incentivize autonomous vehicle progress through a competition and cash prize. Some argue that this race supercharged autonomous vehicle breakthroughs. Although the public history of AI algorithm development is perhaps impressive, one should note that all such policies involve tradeoffs, risks, and implementation challenges. Policymakers should consider the following:
How can the timeliness and efficiency of public research support be improved?
What form of research support, including computational resources, prize challenges, or monetary grants, will best support a given goal?
How might public investments crowd out or supplement private funding? Will such investments distort or privilege certain research outcomes?
How can one best incentivize development while minimizing market distortions?
How can one ensure continued national competitiveness in R&D writ large?
How can algorithms be developed and designed to support principles including democracy, freedom, and fairness?
What types of AI and applications should the public support? Should policy focus on foundational or applied research? For military research, how does one ensure that innovations are designed for dual use?
Open source. A significant portion of AI development is open source, raising questions about safety and regulation and continued innovation. Some worry that open-source, highly capable models will leave potentially harmful software uncontrolled and easily accessible to bad actors. Conversely, some worry that attempts to regulate open source will cost innovation by depressing highly dynamic innovation of the open-source community and weaken the security benefits that transparent, easily critiquable software enjoys. Enforceability is another challenge: how can regulations apply to anonymous actors? Therefore, policymakers should consider the following questions:
What are the costs and benefits of open source AI? Do the cybersecurity and innovation benefits of open-source models outweigh potential risks of open models? What risks do open models pose?
How could any potential regulations be enforced? What are the limits of success?
How can governments improve open-source code? Is there a place for public open-source analysis or vulnerability tracking?
How can public-sector code be open sourced to share potential innovations with other governments, agencies, and actors?
Inputs and Resources
Supply chain robustness. AI chips and hardware require a diverse range of materials and components to support processing needs. A robust AI ecosystem requires supply chains that can reliably source and provision the resources needed by the AI economy. Toward these ends, policymakers should consider the following:
How can the United States trade openly with new markets to ensure access to these goods?
How can the United States ensure an efficient and balanced supply chain?
Can domestic resources help supply the needed materials? How can the United States balance the benefits of domestic resource extraction with environmental costs?
How can the United States ensure access to key resources such as rare minerals? Can alternative materials be developed or discovered to reduce dependence on rare or environmentally harmful materials?
Talent and immigration. AI development requires a range of highly technical and specialized skills. Supporting manufacturing, research, design, and deployment will require a deep talent pool and expensive labor. Education, grants, apprenticeships, and immigration can help fill this gulf. Policymakers should consider the following:
What education policies can incentivize AI and computer science education? What type of skillsets are needed?
How can nontechnical fields be upskilled with AI knowledge to prepare those fields for the potential effect of AI?
Can private-sector incentives for training and apprenticeship programs reduce educational burdens?
How can immigration policy be reformed to attract and retain global talent?
How can AI education balance technical skills with a need for free and creative thinking?
Data resources, privacy, and intellectual property. The scale and source of data often used to train AI systems has prompted a diversity of concerns over data rights, privacy, and intellectual property. Important policy questions include the following:
How can the United States ensure that governments and companies adequately protect the vast and sensitive data used to create their AI systems?
How can the United States mitigate concerns that it will lose an “AI race” to China because authoritarian tools allow for more extensive and detailed data collection?
There is concern that new market entrants with limited data stores and scraping capabilities cannot compete against the vast stores of user data amassed by big tech firms. How can the United States ensure a level playing field and a competitive market?
How can copyright and IP law balance fair use, artistic autonomy, intellectual property rights, and continued AI innovation?
Data standards and interoperability. Data standards can affect the nature and usability of data. Healthcare AI, for instance, has been slow to develop because of highly siloed data, disparate technology practices, and record-keeping differences across systems. A key to this problem is interoperability and standardization. If technology can easily communicate and share data, and if data are standardized and easy to use, this could aid the development of AI systems. Toward these ends, policymakers should consider the following:
How should the government design and format data standards to best serve AI? What information should the data capture? How do these decisions affect the ability to share data, develop AI systems, and promote innovation? Conversely, how might standardization hinder innovation?
How can the government reduce data balkanization to ensure that AI has the tools it needs to grow? How might this be balanced with privacy and security concerns?
How can industry or private actors set and manage standards and data interoperability without government involvement?
Quality Control
Explainability. Because AI systems often focus on capabilities rather than explanation, the reasoning behind their actions can be opaque. Law and policy often require clear reasoning and decision-making. This requirement can raise questions and concerns such as the following:
Should the government risk using autonomous weapons if we do not understand how they select, and possibly kill, targets?
Should the government use AI sentencing algorithms if we do not know if their final decisions are affected by racial biases?
How does the government know that an AI’s decision-making process has not been compromised by a malicious actor?
How might the government know if autonomous vehicles are making safe decisions?
How does the government know that statistical AI models are producing high-quality predictions and results?
Overfitting and underfitting. Overfitting is the problem of fitting a prediction algorithm too tightly to training data, so much so that it underperforms with new data. Underfitting, in turn, is the failure to adequately fit an algorithm to the training data, rendering predictions with new data altogether unreliable. For policy-sensitive applications, AI models must be able to demonstrate that they are neither over- nor underfit for the task at hand. At present, there is no easy solution to this challenge. For policymakers, the best approach is vigilance. The following are examples of issues that this challenge could create:
Economic data have a relatively short history. Treasury models therefore run an underfitting risk that could lead to faulty algorithms when trying to predict inflation, employment, and other key metrics.
Court-sentencing algorithms can run the risk of overfitting. If a case used in a model’s training set is sufficiently unique, the model could carve out a prefabricated decision path that is not generalized but instead is tailored specifically to that set. Should this sort of model, regardless of fitness, ever be used by courts?
Bias and auditing. The data used and the bias embedded in AI algorithms can lead to incorrect or harmful results. AI-powered pulse oximeters, for instance, have been found significantly more inaccurate for dark-skinned patients. Such biases can cause harm. In another case, Amazon found unintentional bias embedded in its hiring algorithm, which favored male applicants far more than female ones. Such biases can cause discrimination. One proposed path forward would be AI audits that could be used to assess algorithmic weaknesses, security, and bias. Regarding bias and auditing, policymakers should consider the following questions to address these issues:
What algorithmic design best practices and industry standards can help spot and mitigate bias?
What data sourcing, cleaning, and processing standards can help minimize bias and ensure robust algorithms? What tradeoffs, unintended consequences, or concerns could such standards create?
Is there an acceptable level of bias? What biases are unacceptable? How does the law deal with AI bias?
Can intentional bias be used to mitigate negative biases? What risks or unintended consequences could this pose?
Should AI audits be required? If so, when and what processes should they include to ensure strong results? Further, would requiring audits place an undue burden on innovation?
Externalities
Energy use, emissions, and environmental impact. Supporting AI requires significant energy use. Chip fabrication requires extensive energy resources, as does the compute-intensive training process. Energy requirements expand as AI algorithms and market demand grow. As a result, intensive computing can leave a high carbon footprint. Cloud computing centers also constrain local energy supplies, potentially increasing local energy prices to support often nonlocal demand. Finally, fabrication produces wastewater and toxic by-products, while cloud computing centers burn through difficult-to-recycle semiconductors. Policymakers should consider the following:
How can the government and private actors balance the energy use and emissions costs of AI systems against the benefits of AI innovation?
Can AI system innovation in energy management and climate research be used to help reduce costs and fight climate change?
What waste and recycling standards and policies can ensure that waste is properly managed?
Labor disruptions. Advances in AI automation may disrupt the workforce and displace certain professions. For instance, in the United States, there are more than 3 million truckers, a generally low-education profession that could be eliminated or transformed by driverless vehicles. Other industries may feel similar strains. Although there is no guarantee that AI will lead to fewer jobs, some people will likely have to find new employment or find that AI changes the nature of their work. As such, policymakers should consider the following:
How can education policy be used to upskill or reskill displaced or under-skilled workers?
How can policy ease workforce transitions and ensure that older workers are not left behind?
How can agencies update or remove regulations that might entrench certain labor classes despite AI automation improvements?
Should the government or private actors ensure redundant human skills in fields automated by AI? If so, how?
Security and Safety
Cybersecurity. The introduction of AI naturally comes with a transformation of the cyberthreat landscape. New threats can be found in AI. The massive scope of certain models can make it difficult to spot vulnerabilities or bad actors. Further, data can act as a new attack surface. Data poisoning attacks seek to inject vulnerabilities into a system through bad data or use data inputs to cause a trained system to malfunction. AI will also be used as a tool of cybersecurity. Offensively, it could be used to hunt and exploit vulnerabilities without human involvement or generate highly convincing spear-phishing emails. Defensively, AI can be used to detect intrusions, spot bugs, and stop bad actors. Policymakers should consider the following:
What processes can be used to detect vulnerabilities not only in algorithms but also in the data and processors that drive these systems?
What standards and best practices can be passed to the private sector to mitigate and minimize AI cyber risks?
How can the government detect and alert the public to systemic AI cyberattacks and risks?
How can the government encourage effective prosocial cybersecurity research and hacking?
How can the government ensure critical infrastructure remains secure and operational?
Supply chain security. The supply chain that supports AI technologies is long, complex, and brittle. Chips are often manufactured abroad, leaving them vulnerable to foreign influence. Data are often collected, sold, and reused. This creates novel threats and attack surfaces. Policymakers should consider the following:
How can the government or private actors gather intelligence about supply chain–based vulnerabilities and threats?
How can the government or private actors detect compromised or counterfeit chips?
How does the government hedge against security threats to its supply chain, such as China’s threat to Taiwan—its primary semiconductor trading partner?
How does the government or private actors balance the need for plentiful resources with the need to minimize the influence of bad actors?
How can the government collaboratively work with its allies to ensure access to safe components?
Content regulation, identification, and moderation. As generative AI grows in quality, and formats such as generated video and audio mature, political scrutiny has grown. When generated content is found obscene, objectionable, or illegal, the content itself is viewed as the problem. In other cases, content use is the challenge. Already AI has been used to generate propaganda, advertisements, and misinformation. Finally, some worry that if AI-generated content isn’t readily identifiable, consumers can be misled. Without the availability of identification tools or procedures, AI-based scams, deep fakes, generated misinformation, and other challenges could easily cause harm. Policymakers might consider the following:
What generated content might be off limits? How might any content restrictions overlap with existing law such as Section 230 of the Communications Decency Act and the First Amendment? How might limits be enforced effectively without harming innovation?
Who is liable for harm related to generated media? What impact would liability questions have on safety, innovation, and deployment?
Should the United States restrict the use of AI-generated materials in certain media, such as advertisements or election materials? If so, how?
How can policymakers respond to generated spam, disinformation, or scams? How can consumers identify AI-generated media? What technologies, rules, or norms are needed to ensure that consumers and governments understand what is generated? Should generated media identification or certain authentication procedures be required?
Lethal autonomous weapons systems. AI algorithms make a reality of robotic weaponry that can select and engage targets without humans in the loop. This is no longer science fiction; such systems are already in use on the battlefield. Policymakers must actively engage in the many now-practical ethical and legal implications of these systems. Questions that policymakers must answer include the following:
How do autonomous weapons conform to international law and the laws of war?
How might arms control law apply to autonomous weapons, and how might the government technically verify a potential arms control agreement?
What role do humans play in controlling or mitigating the potential harms of autonomous weapons?
How can the actions and life-or-death decisions made by autonomous weapons be justified or explained?
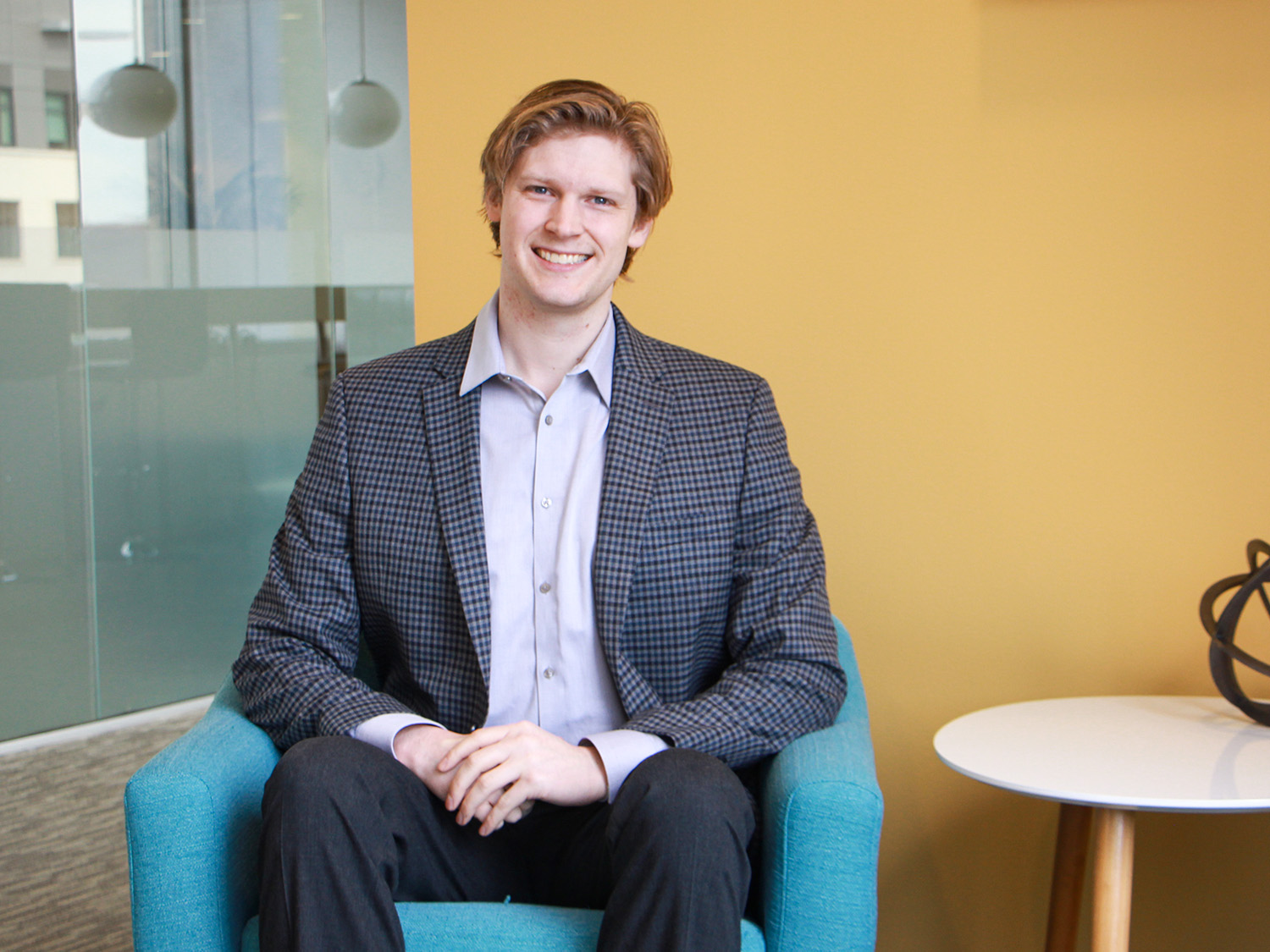